Surgical Artificial Intelligence and Innovation Laboratory
Massachusetts General Hospital
About SAIIL
The Massachusetts General Hospital Surgical Artificial Intelligence and Innovation Laboratory (SAIIL) is a multidisciplinary group composed of surgeons, engineers and data scientists who are passionate about redesigning the delivery of surgical care. The team is made up of surgeons in Mass General’s Department of Surgery and scientists from Massachusetts Institute of Technology Computer Science and Artificial Intelligence Laboratory (CSAIL). Together, our team has developed tools to help unlock the intraoperative phase of care.
Our primary emphasis is on utilizing computer vision to investigate the intraoperative phase of care through real-time, automated surgical analysis. In other words, we use artificial intelligence (AI) to automatically analyze and interpret videos of operations as they are occurring. The goal is to teach the AI to understand what is happening in an operation, determine whether the risk for a postoperative complication is high, or even provide surgeons with additional data to improve operating room decisions.
While the field of surgical research has improved its ability to study pre- and postoperative events and risk using claims data and patient registries, the intraoperative phase of care remains difficult to study.
In a review of nationwide data, researchers estimated that major intraoperative adverse events (i.e. accidental damage to bowel or major blood vessels) can occur in 2% of all operations. Approximately 22 million general surgery operations are performed each year in the United States and 440,000 patients may experience an intraoperative adverse event a year. The cost of hospital admission is 41% higher in these patients, and the consequences of adverse events can impact their quality of life. An expected one-day stay could turn into a month-long hospitalization, additional procedures, prolonged rehabilitation, or a host of other life-altering consequences.
Research Vision and Goals
We will help big data realize personalized medicine for surgical patients. We envision a technology-enabled operating room that pulls data from prior operations for real-time clinical decision making, much like a GPS for surgeons.
We are building technology as the foundation for a worldwide database of surgical cases. A surgeon learns and improves one operation at a time. An AI system can learn from thousands of cases simultaneously. It allows for the collection, analysis and sharing of quantitative evidence in real-time across multiple surgeons—a “collective surgical consciousness.”
The goals of our research in surgery are to:
Democratize surgical knowledge
Lower costs
Improve outcomes
Reduce morbidity and mortality
Research Projects
Our research focuses on using computer analysis to improve operations. By using artificial intelligence, we can work to improve surgical care.
Artificial Intelligence for Risk Prediction from Intraoperative Events
This study will utilize our team's previously developed computer vision-based analysis of intraoperative video to integrate quantitative intraoperative data with perioperative data to improve the prediction of patient-specific complications and readmissions for patients undergoing laparoscopic cholecystectomy.
Funding: CRICO Risk Management Foundation
Automated Intraoperative POEM Analysis: A Machine Learning Approach
The goal of this study is to develop artificial intelligence to generate compact segmentation and summarization of an endoscopic surgical procedure (per oral endoscopy myotomy) in real-time. This study builds off our initial pilot approach utilizing support vector machines for visual classification in sleeve gastrectomy and pivots to the use of deep learning for our visual model.
Funding: Natural Orifice Surgery Consortium for Assessment and Research
Publications
The following are publications from the Mass General SAIIL team:
Caicedo HH, Hashimoto DA, Caicedo JC, Pentland A, Pisano GP. Overcoming barriers to early disease intervention. Nature Biotechnology
Hashimoto DA, Witkowski E, Gao L, Meireles O, Rosman G. Artificial intelligence in anesthesiology: current techniques, clinical applications, and limitations. Anesthesiology. 132(2):379-394.
Fong ZV, Hashimoto DA, Jin G, Haynes AB, et al. Simulated volume-based regionalization of complex procedures: impact on spatial access to care. Ann of Surg.
Hashimoto, Daniel A. MD, MS; Rosman, Guy PhD; Witkowski, Elan R. MD, MPH; Stafford, Caitlin BS; Navarette-Welton, Allison J. BA; Rattner, David W. MD; Lillemoe, Keith D. MD; Rus, Daniela L. PhD; Meireles, Ozanan R. MD: Computer Vision Analysis of Intraoperative Video. Ann Surg. 2019 Sept. DOI: 10.1097
Hashimoto DA, Axelsson C, Jones C, Phitayakom R, Petrusa E, McKinley S, Gee D, Pugh C: Surgical Procedural Map Scoring for Decision-Making in Laparoscopic Cholecystectomy. American Journal of Surgery. 2019 Feb. DOI: https://doi.org/10.1016/j.amjsurg.2018.11.011
Hashimoto DA, Rosman G, Rus DL, Meireles OR. Response: Artificial Intelligence in Surgery Requires Interdisciplinary Collaboration and Understanding. Ann Surg. 2018 Jul 10. PMID: 29995685.
Hashimoto DA, Rosman G, Rus D, Meireles OR. Artificial Intelligence in Surgery: Promises and Perils.Ann Surg. 2018 Jul; 268(1):70-76. PMID: 29389679.
Hashimoto DA, Rosman G, Rus D, Meireles OR. Surgical Video in the Age of Big Data. Ann Surg. 2017 Aug 23. PMID: 28837447.
Volkov M, Hashimoto DA, Rosman G, Meireles OR, Rus D. 2017 IEEE International Conference on Robotics and Automation. Machine learning and coresets for automated real-time video segmentation of laparoscopic and robot-assisted surgery. 2017; 754-759.
Amazing clients have allowed us to produce work we are proud of.
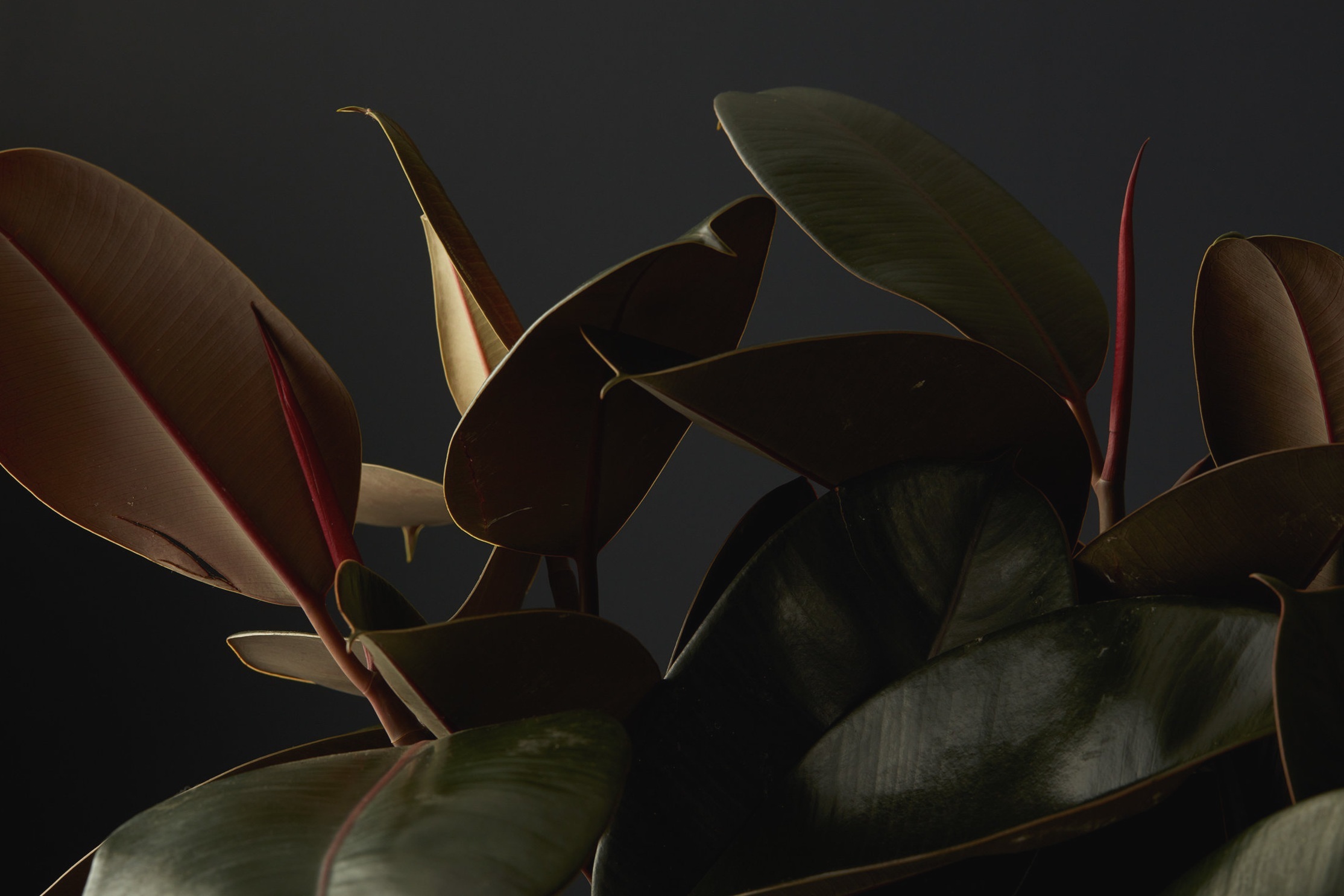
Featured Work
Website design and development for a boutique plant shop in Clinton Hill, New York.